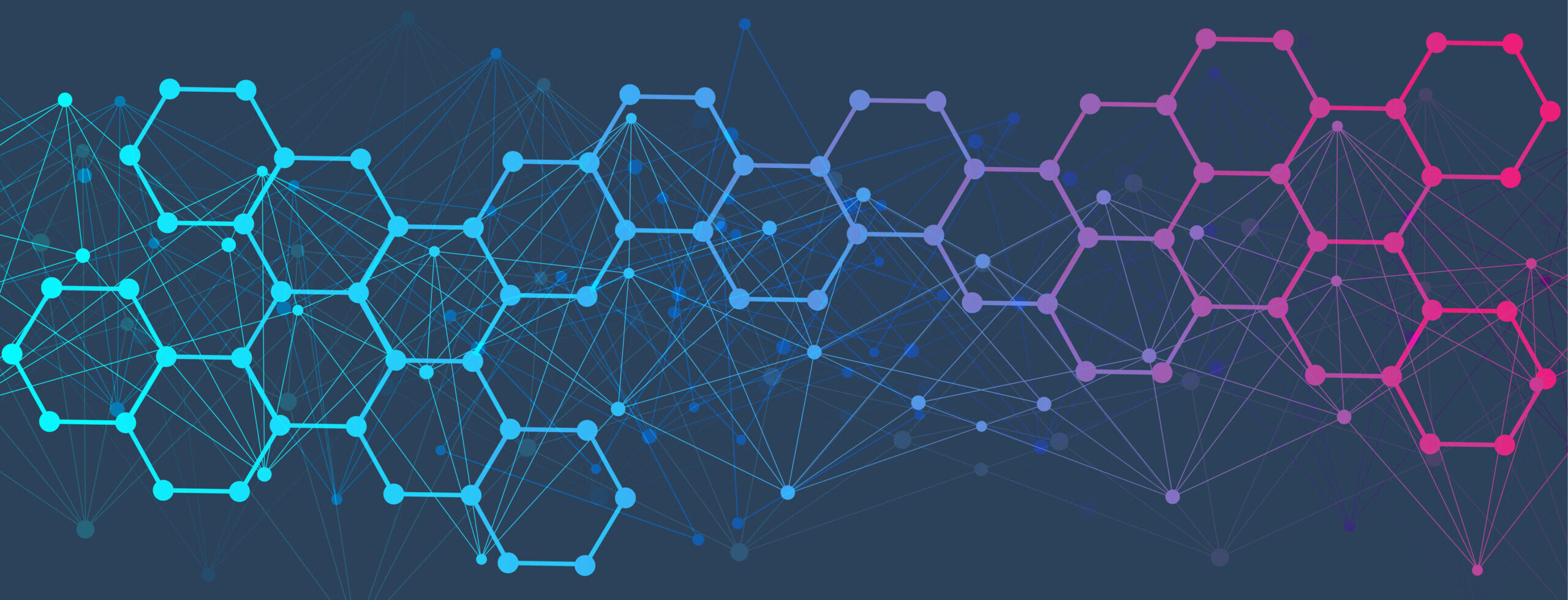
Research
Theme 3: Early Warning Systems of Infectious Diseases
Analysis of early warning signals is a crucial component of a coordinated response to emerging infectious diseases (EIDs). The goal of OMNI-RÉUNIs’ projects is to provide both an analysis of these signals and of the possibility of disease establishment, and collectively these could be used to inform public health regarding the level of disease threat.
Signal Detection from Social Media
Co-Project Investigators: Aijun An, Jude Dzevela Kong and Manos Papagelis (York University)
This project aims to extract features or signals from social media text that are relevant to disease outbreak and at the same time identifying fake signals. We will apply natural language processing techniques, such as linguistic feature extraction, event detection, and deep neural network-based feature extraction, to identify potential signals for disease outbreak. We will investigate machine learning methods for predicting disease outbreak given the identified signals or features.
Digital disease surveillance for Emerging Infectious Diseases
Co-Project Investigator: Zahid Butt (University of Waterloo)
This project aims to analyze people’s internet search behaviour regarding health-related information and social media use to guide real-time surveillance of emerging diseases and help predict epidemics. A digital surveillance system would act as an early warning system and help public health authorities and hospitals to plan and respond to emerging infectious disease threats.
From process to structure of early warning signals
Co-Project Investigators: Frithjof Lutscher (University of Ottawa), Iain Moyles (York University), and Bouchra Nasri (Université de Montréal)
This project aims to study the structure of early warning signals (EWS); it is based on mechanistic models for epidemic and social processes (e.g., disease dynamics, pathogen shedding, doctor’s visits, use of social media, contact network). Model simulations will allow us to study the expected structure of EWS and to quantify the contribution of various processes and network topology to this structure.
Mobility-based models (spatiotemporal) for epidemic spreading, targeted interventions, and predictions
Co-Project Investigator: Manos Paapgelis (York University)
This project emphasizes the need of incorporating heterogeneity in epidemic models based on mobility patterns of individuals. Mobility patterns (captured by Bluetooth or GPS-enabled devices, such as cell phones) allow for accurate tracing of contacts in the community which form the underlying social network on which an epidemic unfolds. Information about the location and time of contacts provides an opportunity to refine traditional epidemiological models, design targeted intervention strategies (as opposed to horizontal measures), and inform better decisions on health policy.
Determining a characteristic structure within multiple early warning signals via machine learning and statistical approaches
Co-Project Investigators: Mark Lewis (University of Victoria), Hao Wang (University of Alberta), Russ Greiner (Univeristy of Alberta) and Pouria Ramazi (Brock University)
This project will use statistical analysis and machine learning to determine a characteristic structure within multiple early warning signals driven by a disease outbreak. More specifically, we will develop machine-learning models to detect the structural signatures in the coordinated surveillance data of EWS1 and to accurately identify the presence of an outbreak. We will then use statistical analyses and machine learning techniques to detect early warnings from objective EWS2. Bayesian networks will be applied to identify key features and reveal the statistical dependencies between the features.
Forecasting new Coronavirus Variants using deep learning
Co-Project Investigator: Hongmei Zhu (York University)
This project analyzes the long-term effects and risk factors of post Covid-19 Symptoms. While most people who were infected by coronavirus disease 2019 (COVID-19) had mild symptoms and recovered fully within a few weeks, recent studies show that 20% of the covid-infected people still suffer at least one long-term health problem months after covid. These post-covid symptoms includes extreme fatigue, lung symptoms, heart problems, and other possible organ damages, etc. We are interested in the use of statistical analysis and machine learning to investigate the possible effects and risk factors of post-covid-19 symptoms to people with infected by the mild version of covid and/or to people with initial chronic diseases.
Mining and Summarization of Early Warning Pandemic Signals for vector-borne diseases (Lyme and Chikungunya, etc)
Co-Project Investigator: Gias Uddin (University of Calgary)
This project aims to develop algorithms and tools to automatically detect early warning signals (EWS) for a pandemic in multiple available data sources like internet activity (e.g., Twitter, Facebook, etick.ca). Social media data and web-scraping are especially effective to detect and understand public sentiment for some infectious diseases (ID). Such detection in texts can be done using advances in Natural Language Processing (NLP) like BERT. However, it is known that domain specificity and contexts play the most crucial role in reliably detecting an ID and its relevant signals in the data sources. We will investigate the design algorithms to detect signals from social media texts, e.g., detection of pandemic-related entities and events, tracing opinions about a particular event across multiple sources, and offering clues of contrastive viewpoint. Once the relevant entities are detected properly, we will create an online dashboard by displaying situation-aware real-time signals.
Network Modelling Approach for Predicting the International and Domestic Spread of Emerging Infectious Disease
Co-Project Investigators: Junling Ma (University of Victoria)
This project aims to develop methods that a) evaluate the risk of case importation into major Canadian cities through international travel; b) detect and give early warnings to domestic spread for cities with imported cases; and c) evaluate the risk of case spread from these to other regions in Canada through domestic travel. When an emerging infectious disease kindles an epidemic outside Canada, it is crucial to estimate the rate that cases land in Canada through international travel, and identity which Canadian cities are at a high risk to see such cases, and the effectiveness of travel restrictions, isolation and quarantine may affect the rate of case importation.
Numerical Modeling and Experimental Validation of Aerosol Transmission in Public Spaces and Public Transportation Modes Considering Different NPI such as Masks vs. Lifted Mask Mandates, and Conventional HVAC vs. Enhanced HVAC Interventions
Co-Project Investigators: Marina Freire-Gormaly (York University)
This project aims to develop validated models for aerosol transmission in public spaces and transportation modes. The project team will analyze the effectiveness of different NPIs, including masks and HVAC interventions, to mitigate the transmission of infectious diseases. In addition, the project will provide insights to inform the development of early warning detection systems for emerging infectious diseases through numerical modelling and experimental validation.